Artificial Ai forms the basis for all computer learning and is the future of all complex decision making
In today’s rapidly evolving technological landscape, artificial intelligence (AI) has emerged as a pivotal force driving innovation across various industries. From healthcare to finance, manufacturing to entertainment, AI applications are ubiquitous, promising to revolutionize the way we work, live, and interact. However, amidst the excitement surrounding AI’s potential, a pertinent question arises: When is artificial intelligence most effective?
To address this question, it’s imperative to delve into the intricacies of AI technology, its capabilities, limitations, and the contextual factors that influence its effectiveness. While AI holds immense promise, its optimal efficacy is contingent upon several key factors, including data quality, problem complexity, human-AI collaboration, and ethical considerations.
Data Quality: The Foundation of AI Effectiveness
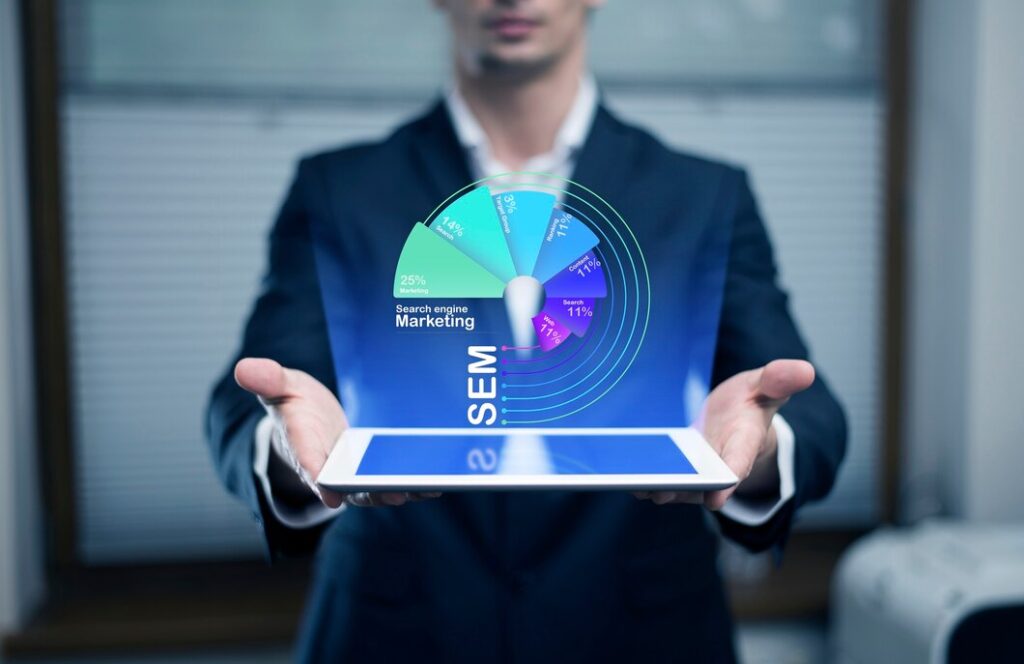
At the heart of AI effectiveness lies the quality of data upon which it operates. AI algorithms rely on vast datasets to learn patterns, make predictions, and generate insights. Consequently, the accuracy, relevance, and representativeness of data significantly influence AI’s performance.
In scenarios where data is abundant, diverse, and accurately labeled, AI excels in tasks such as image recognition, natural language processing, and predictive analytics. For instance, in medical diagnostics, AI algorithms trained on comprehensive patient datasets can detect anomalies with remarkable accuracy, aiding physicians in early disease detection and personalized treatment planning.
Conversely, in domains where data is scarce, noisy, or biased, AI effectiveness diminishes. Biased datasets can perpetuate discriminatory outcomes, exacerbating societal inequalities and undermining trust in AI systems. Therefore, ensuring data quality through rigorous curation, bias mitigation, and transparency measures is paramount for maximizing AI effectiveness across diverse applications.
Problem Complexity: Matching AI Capabilities with Task Demands

Another crucial determinant of AI effectiveness is the complexity of the problem at hand. AI excels in tasks characterized by pattern recognition, optimization, and decision-making under uncertainty. However, its effectiveness varies across different problem domains, depending on factors such as task complexity, ambiguity, and context specificity.
In highly structured domains with well-defined rules and objectives, such as game playing or logistics optimization, AI algorithms like AlphaGo or route optimization algorithms demonstrate remarkable effectiveness, surpassing human performance in certain tasks. Conversely, in unstructured environments with ambiguous goals and evolving dynamics, such as creative design or strategic decision-making, AI’s effectiveness may be limited, requiring human intuition, creativity, and contextual understanding to complement algorithmic capabilities.
Moreover, the interdisciplinary nature of complex problems often necessitates hybrid approaches that integrate AI with human expertise. Collaborative AI systems, where humans and algorithms work synergistically, leverage the strengths of both parties to tackle intricate challenges more effectively. For instance, in cybersecurity operations, AI-powered threat detection systems can augment human analysts by identifying suspicious patterns and anomalies, enabling timely response and mitigation strategies.
Human-AI Collaboration: Harnessing Synergy for Enhanced Performance
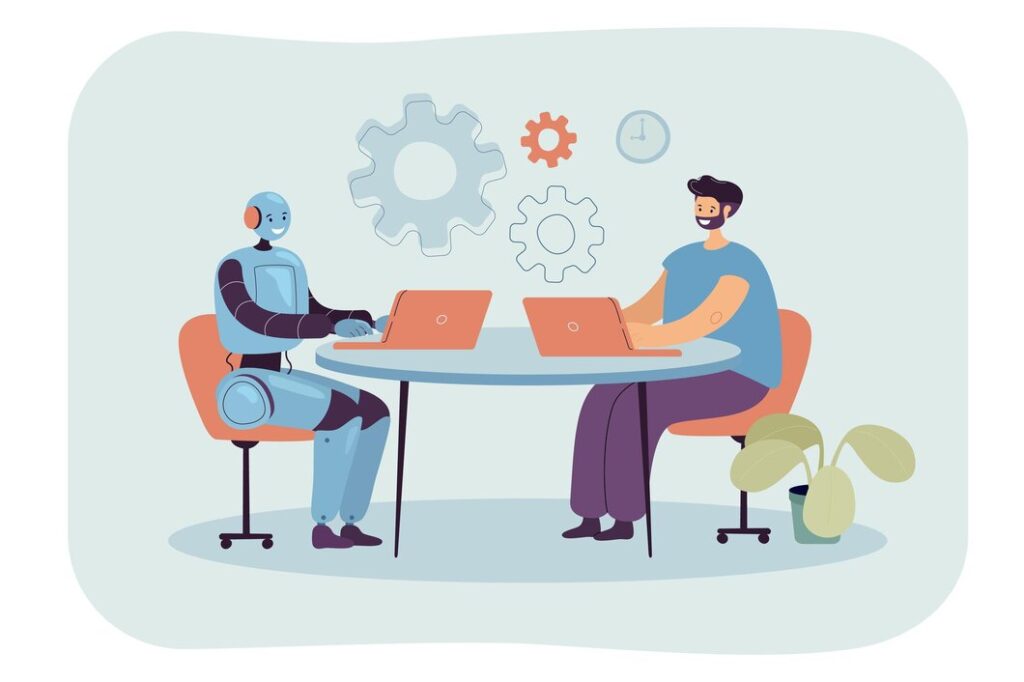
In the era of human-centric AI, the role of human-AI collaboration emerges as a critical factor shaping AI effectiveness. Rather than viewing AI as a replacement for human labor, organizations are increasingly embracing a collaborative paradigm where humans and machines complement each other’s strengths, mitigate weaknesses, and amplify overall performance.
Effective human-AI collaboration requires seamless integration of AI systems into existing workflows, transparent communication of AI-generated insights, and mechanisms for feedback and adaptation. By leveraging AI to automate routine tasks, augment human decision-making, and uncover hidden patterns in data, organizations can unlock new levels of productivity, innovation, and agility.
For instance, in customer service operations, chatbots powered by natural language processing can handle routine inquiries and transactional tasks, freeing up human agents to focus on complex issues requiring empathy and creative problem-solving. Similarly, in scientific research, AI algorithms can analyze vast datasets, identify research trends, and generate hypotheses, empowering scientists to accelerate discoveries and breakthroughs in diverse fields.
Ethical Considerations: Safeguarding AI’s Societal Impact
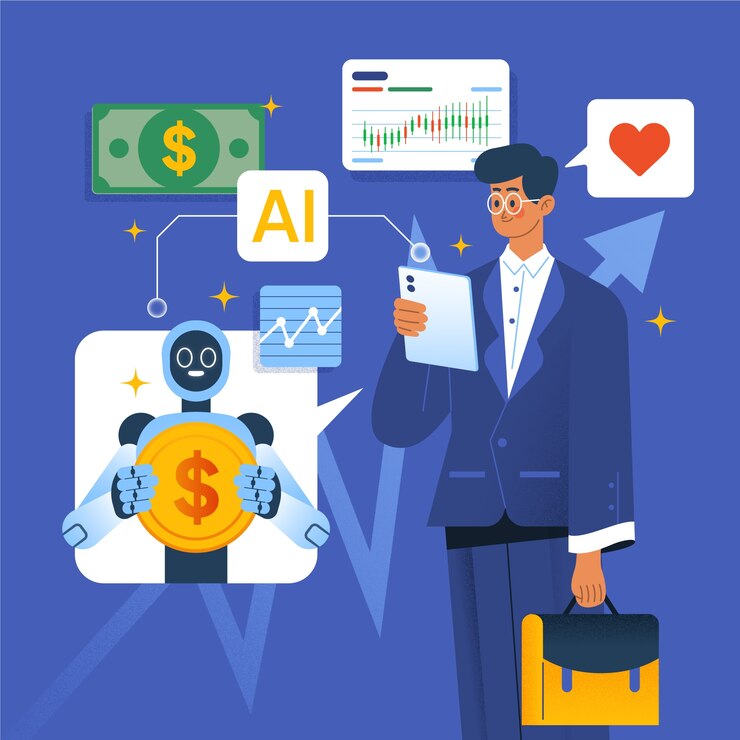
As AI permeates various facets of society, ethical considerations loom large in discussions surrounding its effectiveness. Ensuring that AI is deployed responsibly, ethically, and equitably is essential for fostering trust, mitigating risks, and maximizing its societal benefits.
Key ethical considerations in AI deployment include fairness and bias mitigation, transparency and explainability, privacy and data protection, accountability and liability, and societal impact assessment. By embedding ethical principles into the design, development, and deployment of AI systems, stakeholders can mitigate unintended consequences, address societal concerns, and foster inclusive, human-centered AI ecosystems.
For instance, in algorithmic decision-making systems, proactive measures to mitigate biases and ensure fairness are paramount to prevent discriminatory outcomes and promote equal opportunities. Likewise, transparent AI systems that provide users with insights into decision-making processes and underlying data sources enhance trust, accountability, and user acceptance.
Things You Should Know About Artificial AI
In conclusion, the effectiveness of artificial intelligence is shaped by a myriad of interrelated factors, including data quality, problem complexity, human-AI collaboration, and ethical considerations. By understanding these dynamics and navigating the complexities inherent in AI deployment, organizations can harness the transformative potential of AI to drive innovation, enhance productivity, and address societal challenges effectively.
However, realizing the full benefits of AI requires a holistic approach that balances technological advancements with ethical imperatives, human values, and societal needs. By fostering interdisciplinary collaboration, promoting transparency and accountability, and prioritizing human well-being, we can harness the power of AI as a force for positive change, driving progress and prosperity in the digital age.
1 thought on “When Is Artificial AI Most Effective”